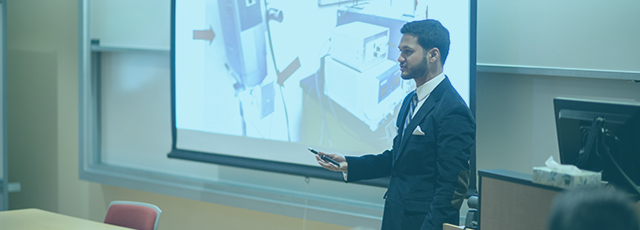
Oral Presentations
Document Type
Union College Only
Faculty Sponsor
Nicholas Webb
Department
Computer Science
Start Date
22-5-2020 10:30 AM
Description
Recent developments in natural language understanding (NLU) research have given rise to pre-trained language models for contextualized word representations. XLNet, the most recent iteration of these generalized language models, advances the state-of-the-art on multiple benchmarks, outperforming its highly successful predecessor BERT on 18 of 20 language understanding tasks. Several successful attempts at adapting BERT for both extractive and abstractive text summarization have recently been proposed. In this study, we leverage XLNet for the task of extractive summarization by stacking and jointly fine-tuning a sentence-level classification layer on output representations for sentence selection. Results on the CNN/DailyMail text summarization dataset are competitive with the state-of-the-art with an architecture that has fewer trainable parameters than other approaches.
Pre-Trained Contextualized Word Representations for Extractive Text Summarization
Recent developments in natural language understanding (NLU) research have given rise to pre-trained language models for contextualized word representations. XLNet, the most recent iteration of these generalized language models, advances the state-of-the-art on multiple benchmarks, outperforming its highly successful predecessor BERT on 18 of 20 language understanding tasks. Several successful attempts at adapting BERT for both extractive and abstractive text summarization have recently been proposed. In this study, we leverage XLNet for the task of extractive summarization by stacking and jointly fine-tuning a sentence-level classification layer on output representations for sentence selection. Results on the CNN/DailyMail text summarization dataset are competitive with the state-of-the-art with an architecture that has fewer trainable parameters than other approaches.