Files
Download Full Text (274 KB)
Document Type
Union College Only
Faculty Sponsor
Jue Wang
Department
Mathematics
Description
Kidney stone (KS) disease is the most common disease of the urinary tract. Over 1.3 million individuals visit emergency departments (ED) for stone-related symptoms, which is projected to increase. Since most lab tests are misinterpreted, leading to an incorrect diagnosis, diagnostic predictive tools are useful to most accurately calculate an individual's likelihood, or risk, of having KS disease. We investigate which predictive model would yield the highest accuracy and sensitivity by extracting information from a clinical database containing patient data and utilize various machine learning and statistical analysis to analyze the performance of each model. With using confusion matrices, logistic regression, and ROC curves, it has been found that including all domains in a predictive model (demographics, laboratory tests, ICD-9 diagnoses, etc.) demonstrated the highest performance.
Recommended Citation
Atkins, Ally, "Predicting Kidney Stone Disease Through Models and Machine Learning" (2020). 2020 Summer Research Poster Session. 17.
https://digitalworks.union.edu/srps_2020/17
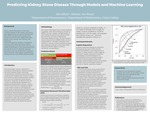
Comments
Detecting kidney stones, especially in emergency departments, requires multiple lab tests (complete blood count with comprehensive metabolic panel and urine analysis) and imaging tests. However, These test results are often misinterpreted, leading to an incorrect diagnosis and prognosis. Therefore, diagnostic predictive tools are useful for calculating the likelihood of a patient developing kidney stones., such as STONE PLUS. However, significant efforts can be made to improve a KS diagnostic model, such as incorporating laboratory data and additional clinical characteristics. Creating an improved KS predictive tool would lead to earlier diagnosis and decrease the number of unnecessary imaging, thereby reducing the costs. We investigate which predictive model performs best using machine learning and statistical analysis.